The U.S. tech industry is grappling with a significant gender gap. The disparity is stark with only 14% of tech leaders being women and the number of women dropping as the career level rises. Recent moves by tech giants like Zoom and Meta to scale back their DEI (Diversity, Equity, and Inclusion) efforts only exacerbate these challenges, threatening to deepen systemic barriers to equal opportunities.
As AI becomes increasingly integrated into employee selection processes, the importance of how these systems are trained cannot be overstated. Depending on its development and implementation, AI has the potential to either perpetuate existing biases or become a powerful tool for promoting diversity and inclusion. Without careful consideration and inclusion of diverse perspectives in AI development, the risk of reinforcing power imbalances for women and minorities is substantial.
The Role of AI in Employee Selection
AI is widely implemented to automate selection processes to increase efficiency and objectivity. However, if AI models are trained on biased data, they can inadvertently perpetuate existing disparities. This highlights the critical need for companies to ensure that their AI training processes are objective and inclusive.
Reducing DEI initiatives risks losing diverse talent, damages company culture, and invites backlash. As we face challenges in maintaining diversity in the workforce, organizations must prioritize DEI efforts.
The decline in diversity among tech professionals underscores the importance of sustained DEI efforts. Organizations must improve communication about DEI initiatives and foster transparency to build understanding and buy-in. Equally important is ensuring that AI is not biased through the use of non-diverse training data.
Benefits of Diverse Teams
Diverse teams are shown to be 87% more likely to make better decisions, and inclusive companies experience a 2.3x increase in cash flow per employee. These statistics underscore the tangible benefits of fostering diversity within organizations.
The most significant issue for improving diversity is how AI models are trained. Companies must ensure their models are genuinely objective. AI itself shouldn’t have personal biases, but it will if the models use biased data.
The DEI issue will not be solved in one year. It will take many years, and companies will make mistakes if the effort is rushed. We must continue to champion diversity and inclusion to drive innovation and success.
Improving AI Training
To mitigate gender disparities in hiring and support DEI initiatives, companies need to focus on several key areas:
- Diverse Training Data: AI models should be trained on diverse datasets that reflect various backgrounds and experiences. This helps prevent the perpetuation of biases inherent in historical hiring practices.
- Bias Mitigation Techniques: Implementing techniques to detect and mitigate biases in AI models is crucial. This can include regular audits of AI systems and incorporating fairness constraints into model training.
- Inclusive Development Teams: Having diverse AI development teams can provide varied perspectives, helping to identify and address potential biases in AI systems. The curb-cut effect, where designing for inclusivity benefits everyone, is relevant.
- Ethical Considerations: Ethical considerations must be central to AI development and deployment. This includes transparency in AI decision-making processes and accountability for the outcomes produced by AI systems.
The Impact of Biased AI Models
Biased AI models can perpetuate gender disparities by reinforcing stereotypes and unfairly disadvantaging certain groups. If AI models are trained on historical recruitment data, they will likely replicate the biases inherent in those datasets.
Traditional hiring practices have often favored specific backgrounds and characteristics, inadvertently sidelining candidates from diverse or non-traditional backgrounds. For example, a model that heavily emphasizes a candidate’s alma mater might overlook highly talented individuals who did not attend prestigious institutions. Instead, training AI models with data from successful candidates of diverse backgrounds can help identify and recruit talent that might otherwise go unnoticed.
AI can more objectively assess candidates by focusing on skills and responses to specific questions rather than educational background or other potentially biased criteria. This approach aligns with the goal of building models that prioritize relevant qualifications over subjective experiences. Such models help create a level playing field, ensuring all candidates have a fair chance regardless of their background.
Leveraging AI for DEI
AI can be leveraged to enhance diversity and inclusion efforts by:
- Identifying Biases: AI can help identify biases in current recruitment practices, highlighting areas where improvements are needed.
- Supporting Objective Decision-Making: AI can standardize and objectify hiring criteria, reducing the influence of subjective human biases.
- Promoting Underrepresented Groups: AI can be programmed to actively seek out and promote candidates from underrepresented groups, ensuring a more diverse talent pool.
To further explore the importance of unbiased AI training in employee selection processes, consider in what ways biased AI models perpetuate gender disparities in hiring processes, and what role do diverse perspectives play in developing and deploying AI technologies.
Companies should also ask how can improved AI training in employee selection processes help bridge the gender gap in the tech industry. AI should be leveraged to enhance, rather than hinder, diversity and inclusion efforts within tech organizations. That’s why organizations must investigate the potential consequences of not integrating diversity into AI development teams and ask how they can ensure that AI algorithms used in a fair and unbiased way in hiring. Finally, look at the ways can AI be leveraged to identify and address systemic barriers to gender equality in tech careers.
By addressing these issues, companies can better understand the complexities of AI and DEI and work towards creating more inclusive and equitable workplaces. Improving AI training in employee selection processes is not just a technological challenge but a critical step towards building diverse teams, fostering innovation, and upholding DEI initiatives in the tech industry.
Explore HRTech News for the latest Tech Trends in Human Resources Technology.
ABOUT THE AUTHOR
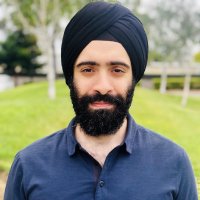
Kiranbir Sodhia
AI at Google
Kiranbir Sodhia, a distinguished leader and engineer in Silicon Valley, California, has spent over 15 years at the cutting edge of AI, AR, gaming, mobile app, and semiconductor industries. His expertise extends beyond product innovation to transforming tech teams within top companies. At Microsoft, he revitalized two key organizations, consistently achieving top workgroup health scores from 2017 to 2022, and similarly turned around two teams at Google, where he also successfully mentored leaders for succession. Kiranbir’s leadership is characterized by a focus on fixing cultural issues, nurturing talent, and fostering strategic independence, with a mission to empower teams to operate independently and thrive.